
An illustration of AI integrating with telemedicine platforms to enhance patient care.
In today’s world, where technology is part of everything we do, the combination of telemedicine and AI is truly transforming how healthcare is delivered. This powerful partnership isn’t just making healthcare more accessible, it’s also allowing us to personalize care and even predict patient health trends in ways we haven’t seen before. In this piece, I’ll take a closer look at how AI and machine learning are enhancing telemedicine platforms, helping us anticipate health patterns, and tailoring care to individual patients.
The Evolution of Telemedicine
Telemedicine has reshaped how we think about healthcare, making it possible to provide clinical services remotely through digital platforms. What started as a way to offer care to people in remote locations has now evolved into a global healthcare solution. With high-speed internet, smartphones, and connected devices becoming widespread, telemedicine has quickly grown into a vital part of today’s healthcare system.
A Look Back
In its early days, telemedicine was pretty basic, limited to phone calls and simple telehealth services. But with advancements in technology, its capabilities have expanded dramatically. Today, we see video consultations, remote monitoring tools, and mobile health apps delivering a wide range of services, from mental health support to chronic disease management, and even complex procedures like remote surgeries using robotics.
How the COVID-19 Pandemic Changed Everything
COVID-19 played a huge role in accelerating telemedicine's growth. With social distancing and reduced hospital visits, telehealth services surged. McKinsey & Company reported that telehealth usage stabilized at levels 38 times higher than pre-pandemic numbers. This boom in adoption showcased the real potential of telemedicine to provide effective care without the need for physical visits, keeping both patients and providers safer.
Where We Stand Now
Telemedicine platforms today offer a broad array of services, from straightforward consultations to advanced diagnostic tools. Wearable tech and health apps now allow continuous monitoring of vital signs in real time. This easy access to care empowers patients to take control of their health while also eliminating the barriers that used to limit access to quality healthcare, such as geography and cost.
The Power of AI in Healthcare
AI and machine learning algorithms have emerged as powerful tools in healthcare, capable of analyzing vast amounts of data to identify patterns, make predictions, and support decision-making processes. The integration of AI into telemedicine platforms significantly enhances their capabilities, offering predictive insights and personalized care plans that were previously unattainable.
Diagnostic Accuracy and Speed
AI algorithms excel at processing complex datasets, such as medical images and genomic information. In radiology, for instance, AI-powered tools can detect abnormalities in imaging studies faster and sometimes more accurately than human clinicians. This capability reduces diagnostic errors and accelerates treatment initiation, improving patient outcomes.
Resource Optimization
Healthcare systems often face challenges related to resource allocation, such as staffing shortages and limited equipment availability. AI can optimize scheduling, predict patient influx, and manage supply chains more effectively. By anticipating needs, healthcare providers can allocate resources where they are most needed, enhancing operational efficiency.
Data Integration and Interoperability
One of the significant hurdles in healthcare is the siloed nature of data across different platforms and institutions. AI facilitates data integration from various sources, including electronic health records (EHRs), laboratory systems, and patient-reported outcomes. This interoperability ensures that clinicians have a comprehensive view of a patient's health status, leading to better-informed decisions.
Enhancing Telemedicine Platforms
When integrated with telemedicine, AI can automate routine tasks such as appointment scheduling, billing, and even preliminary patient assessments using chatbots. This automation allows healthcare professionals to focus more on patient care rather than administrative duties. Moreover, AI-driven analytics provide insights into patient populations, helping organizations tailor services to meet specific community needs.
Predicting Patient Health Trends with AI
Data-Driven Insights
By leveraging electronic health records, wearable device data, and patient-reported information, AI algorithms can predict health trends with remarkable accuracy. The use of big data analytics enables the identification of patterns and correlations that are not apparent through traditional analysis methods.
Chronic Disease Management
For chronic diseases such as diabetes, hypertension, and chronic obstructive pulmonary disease (COPD), continuous monitoring is essential. AI algorithms analyze data from glucose monitors, blood pressure cuffs, and spirometers to detect deviations from normal patterns. For example, AI can predict a potential hypertensive crisis by recognizing subtle increases in blood pressure trends over time.
Behavioral Health Predictions
Mental health is another area where AI shows promise. By analyzing speech patterns, facial expressions during video consultations, and interaction data from apps, AI can identify early signs of depression or anxiety disorders. This proactive approach enables timely interventions, which are critical in mental health management.
Public Health Surveillance
On a larger scale, AI can aggregate anonymized data to identify public health trends. During infectious disease outbreaks, AI models can predict hotspots and the spread of illness based on data from telemedicine consultations and social media. This information is invaluable for public health officials in deploying resources effectively.
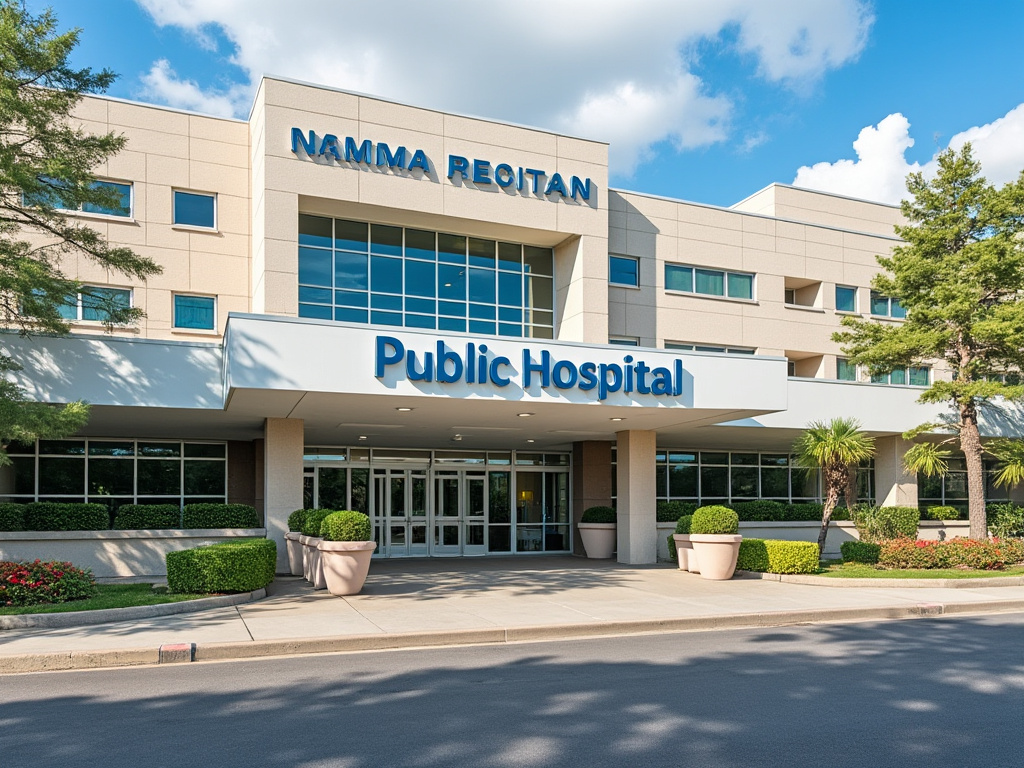
Early Detection and Intervention
Predictive analytics enable early detection of diseases, often before clinical symptoms become apparent. This capability is transformative, shifting healthcare from a reactive to a proactive model.
Cancer Detection
AI algorithms have shown proficiency in detecting early signs of cancers, such as melanoma and breast cancer, through image analysis. For instance, AI can analyze dermoscopic images to identify malignant lesions with high accuracy. Early detection significantly increases the chances of successful treatment and survival.
Cardiovascular Risk Assessment
By analyzing data such as lipid profiles, blood pressure readings, and lifestyle factors, AI can calculate an individual's risk of cardiovascular events. Patients identified as high-risk can receive targeted interventions, such as medication adjustments and lifestyle counseling, to mitigate these risks.
Remote Monitoring and Alerts
Wearable devices equipped with AI can monitor patients in real-time and send alerts to healthcare providers if abnormal readings are detected. For example, an AI-powered heart monitor can notify a cardiologist if a patient experiences arrhythmias, allowing for immediate action.
A study published in the Journal of Medical Internet Research demonstrated how AI could predict hospitalization risks in patients with chronic diseases using telehealth data. The study highlighted the potential of AI in reducing hospital admissions and improving patient quality of life.
Personalizing Care Plans with Machine Learning
Tailored Treatment Strategies
Machine learning algorithms analyze individual patient data to create personalized care plans. This personalized approach considers genetic makeup, lifestyle, environmental factors, and preferences, leading to more effective treatments.
Pharmacogenomics
AI can process genomic data to predict how a patient will respond to certain medications. This information helps clinicians select the most effective drugs with the fewest side effects, a practice known as precision medicine. For instance, in oncology, AI assists in choosing targeted therapies based on the genetic profile of both the patient and the tumor.
Lifestyle and Behavioral Modifications
By analyzing data on diet, exercise, sleep patterns, and stress levels, AI can provide personalized recommendations to improve health outcomes. For example, AI can design a nutrition plan tailored to a patient's metabolic needs and dietary preferences, enhancing adherence and effectiveness.
Rehabilitation Programs
In physical therapy, AI can create customized rehabilitation exercises based on the patient's progress and feedback. Virtual coaches powered by AI provide real-time guidance and adjustments to exercises, optimizing recovery.
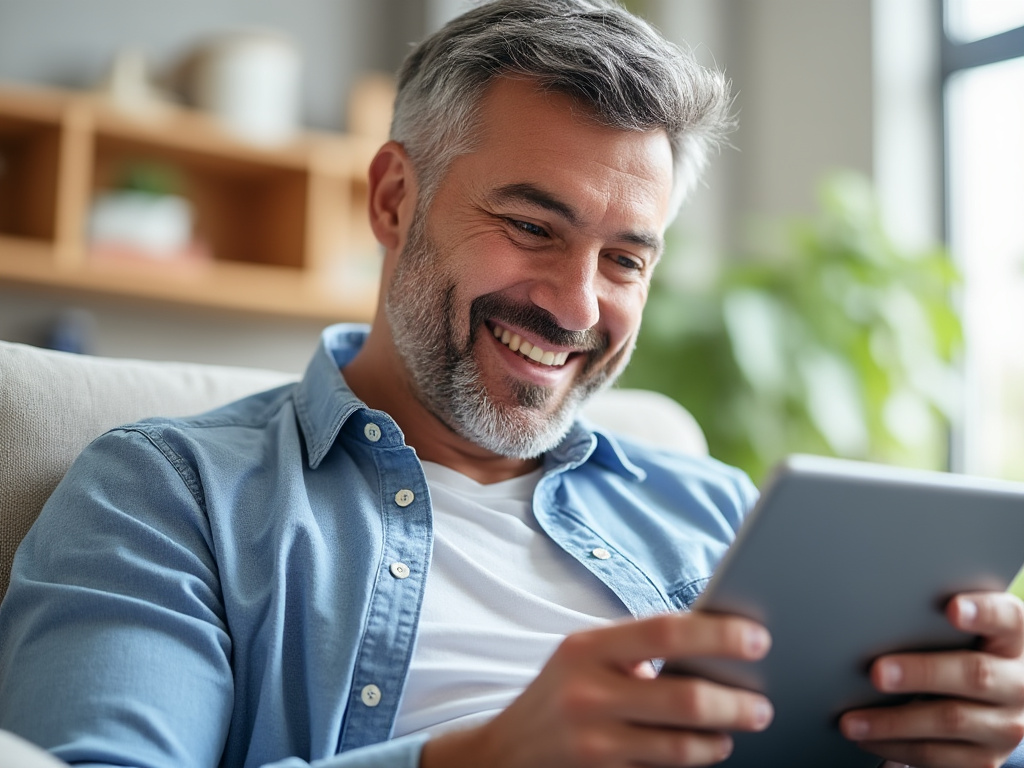
Enhancing Patient Engagement
Personalized care plans increase patient engagement by making healthcare more relevant to the individual. AI-driven tools facilitate continuous interaction between patients and healthcare providers.
Virtual Health Assistants
AI-powered virtual assistants interact with patients through voice or text, answering questions, providing health education, and reminding them of appointments and medication schedules. This continuous engagement keeps patients informed and involved in their care.
Gamification and Motivation
AI can incorporate gamification elements into health apps, encouraging patients to achieve health goals through rewards and challenges. This approach has been effective in promoting behaviors like increased physical activity and medication adherence.
Feedback and Adaptation
Machine learning models adapt to patient behaviors over time. If a patient is not responding to a particular intervention, AI can adjust the care plan accordingly. This dynamic adaptation ensures that the care plan remains effective and aligns with the patient's changing needs.
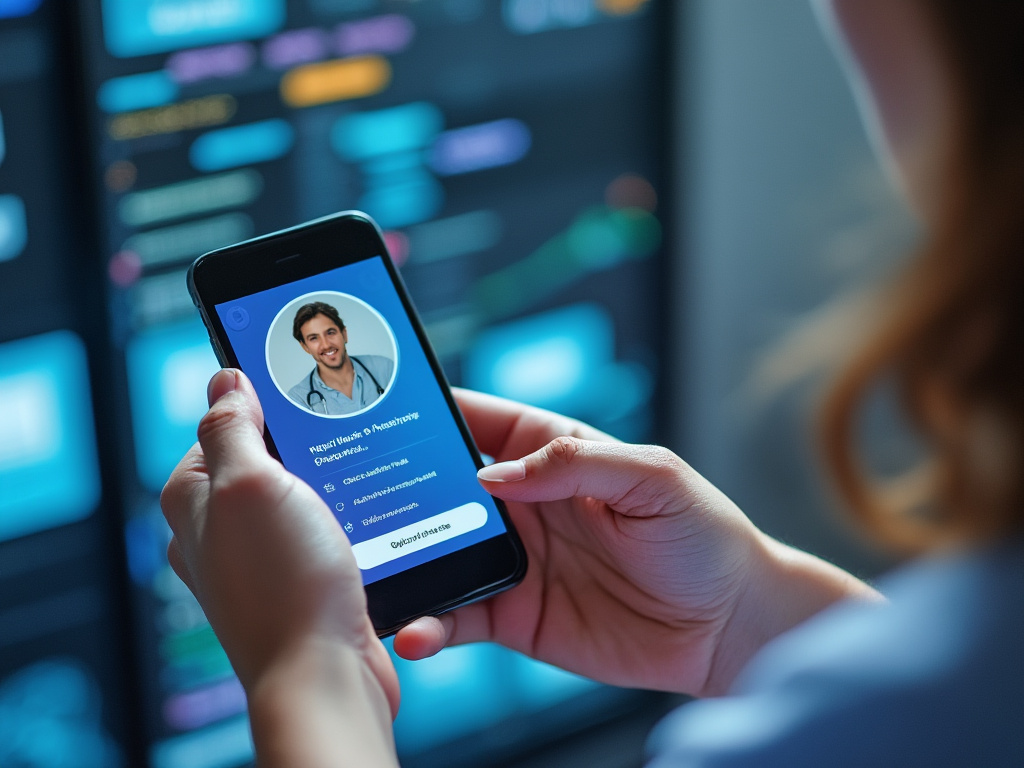
Benefits of AI-Enhanced Telemedicine
- Improved Patient Outcomes: Early detection and personalized care lead to better health outcomes. Patients receive interventions tailored to their specific needs, which enhances the effectiveness of treatments.
- Increased Efficiency: Automation of routine tasks allows healthcare providers to focus on complex cases. AI streamlines workflows, reducing administrative burdens and minimizing errors associated with manual processes.
- Cost Savings: Predictive analytics can reduce hospital readmissions and unnecessary tests, saving costs for both providers and patients. Efficient resource utilization leads to overall cost reductions in the healthcare system.
- Accessibility: Telemedicine bridges the gap for patients in remote areas, and AI ensures they receive high-quality care. AI-powered tools can operate 24/7, providing support whenever patients need it.
- Enhanced Clinical Decision Support: AI provides clinicians with evidence-based recommendations, supporting diagnostic and therapeutic decisions. This support can be particularly valuable in complex cases or when dealing with rare conditions.
- Patient Empowerment: By providing patients with tools and information, AI enhances patient autonomy. Empowered patients are more likely to engage in preventive behaviors and adhere to treatment plans.
Challenges and Considerations
Data Privacy and Security
With the increased use of patient data, ensuring privacy and security is paramount. Cybersecurity threats pose significant risks to sensitive health information.
Regulatory Compliance
Compliance with regulations like the Health Insurance Portability and Accountability Act (HIPAA) in the United States and the General Data Protection Regulation (GDPR) in the European Union is essential. These regulations mandate strict controls over data access, storage, and sharing.
Encryption and Security Measures
Implementing advanced encryption methods and secure authentication protocols protects data during transmission and storage. Regular security audits and vulnerability assessments help identify and mitigate potential risks.
Patient Consent and Transparency
Patients must be informed about how their data is used. Obtaining informed consent and providing options for patients to control their data enhances trust in AI-powered telemedicine solutions.
Ethical Implications
AI algorithms must be transparent and free from bias. There is a need for ethical guidelines to govern AI use in healthcare to prevent disparities in care.
Algorithmic Bias
If AI models are trained on biased data, they may perpetuate or exacerbate existing health disparities. Ensuring diversity in training datasets and regularly evaluating algorithms for bias is crucial.
Accountability and Transparency
Clinicians and patients should understand how AI algorithms make decisions. Explainable AI techniques help make the decision-making process transparent, which is essential for clinical acceptance and ethical responsibility.
Patient Autonomy
While AI can provide recommendations, the final decision should rest with the patient and healthcare provider. Respecting patient autonomy and providing options ensures ethical care delivery.
Technical Limitations
Implementing AI in telemedicine requires robust infrastructure and technical expertise. Integration challenges may arise with existing systems.
Interoperability Issues
Healthcare systems often use a variety of software and platforms that may not communicate effectively. Developing interoperable solutions that can seamlessly integrate with existing EHRs and telemedicine platforms is essential.
Scalability
As the volume of data grows, AI systems must scale accordingly. Ensuring that infrastructure can handle increased loads without compromising performance is a technical challenge.
User Training
Healthcare providers and patients need training to effectively use AI-enhanced telemedicine tools. Investing in education and support resources is necessary to maximize the benefits of these technologies.
The Future of Predictive Telemedicine
The integration of AI in telemedicine is still evolving. Future developments may include:
- Advanced Predictive Models: Utilizing genomics, proteomics, and advanced biomarkers for even more precise predictions. AI could integrate multi-omics data to predict disease susceptibility and drug responses with unprecedented accuracy.
- Integration with Internet of Medical Things (IoMT): Seamless connectivity between devices for real-time monitoring and data collection. IoMT devices, ranging from smart implants to environmental sensors, will provide comprehensive data for AI analysis.
- Enhanced Virtual Reality (VR) and Augmented Reality (AR) Applications: Using VR and AR for remote diagnostics, surgical training, and therapy. Clinicians could perform virtual examinations, and patients could receive immersive therapy experiences for conditions like phobias or chronic pain.
- Blockchain for Data Security: Incorporating blockchain technology to enhance data security and integrity. Decentralized data storage and immutable records could address many privacy concerns.
- AI-Driven Clinical Trials: Accelerating drug development by identifying suitable candidates and predicting outcomes. AI can streamline the recruitment process and monitor participants remotely.

Conclusion
The integration of AI with telemedicine platforms heralds a new era in healthcare. By predicting patient health trends and personalizing care plans, AI-enhanced telemedicine has the potential to improve outcomes, increase efficiency, and make healthcare more accessible. While challenges exist, particularly in data privacy, ethical considerations, and technical implementation, the benefits are substantial. As we navigate these challenges and embrace the innovations, the future of healthcare looks promising, with AI and telemedicine at the forefront of this transformation.
References:
- McKinsey & Company. (2021). Telehealth: A quarter-trillion-dollar post-COVID-19 reality? Retrieved from https://www.mckinsey.com/industries/healthcare-systems-and-services/our-insights/the-virtual-healthcare-imperative
- Journal of Medical Internet Research. (2020). Predicting Hospitalization at Home: Development and Validation of a Machine Learning Model Using Telehealth Data. Retrieved from https://www.jmir.org/2020/11/e20791/
Keywords: Telemedicine, Artificial Intelligence, AI in Healthcare, Predictive Healthcare, Machine Learning, Personalized Care Plans, Health Trends Prediction, Telemedicine Platforms, Remote Patient Monitoring, Healthcare Technology, Digital Health, Telehealth, AI Algorithms, Patient Engagement, Healthcare Innovation, Data Privacy in Healthcare, Ethical AI, Internet of Medical Things (IoMT), Virtual Health Assistants, Future of Healthcare
Related posts
The operating system for value-based care
And experience the impact of telemedicine within your organisation
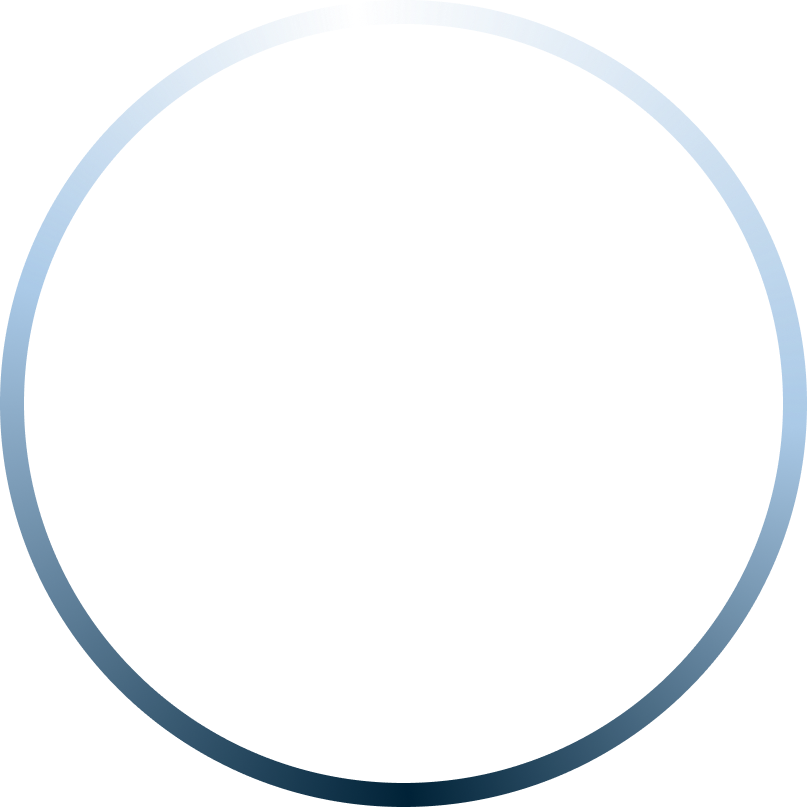